Recently Colleen and Brad had a debate about whether Medical Device Reports (“MDRs”) tend to trail recalls, or whether MDRs tend to lead to recalls. Both Colleen and Brad have decades of experience in FDA regulation, but we have different impressions on that topic, so we decided to inform the debate with a systematic look at the data. While we can both claim some evidence in support of our respective theses based on the analysis, Brad must admit that Colleen’s thesis that MDRs tend to lag recalls has the stronger evidence. We are no longer friends. At the same time, the actual data didn’t really fit either of our predictions well, so we decided to invite James onto the team to help us figure out what was really going on. He has the unfair advantage of not having made any prior predictions, so he doesn’t have any position he needs to defend.
Results
Before showing the actual data analysis, just to keep Brad humble, we started with his theoretical prediction of what a well-functioning system would show, i.e., that a ramping up of MDRs leads to a recall, and the conduct of the recall then leads to a decline in MDRs as the recall becomes effective. Theoretically, Brad was positing that we would see essentially a bell curve with zero, or the highest point, being the date the recall is initiated. If we all lived in fictional Lake Wobegon where life is perfect and "all the children are above average," pictorially, theory would suggest we would see something like this, where the y-axis (which is mostly covered) represents the number of MDRs:
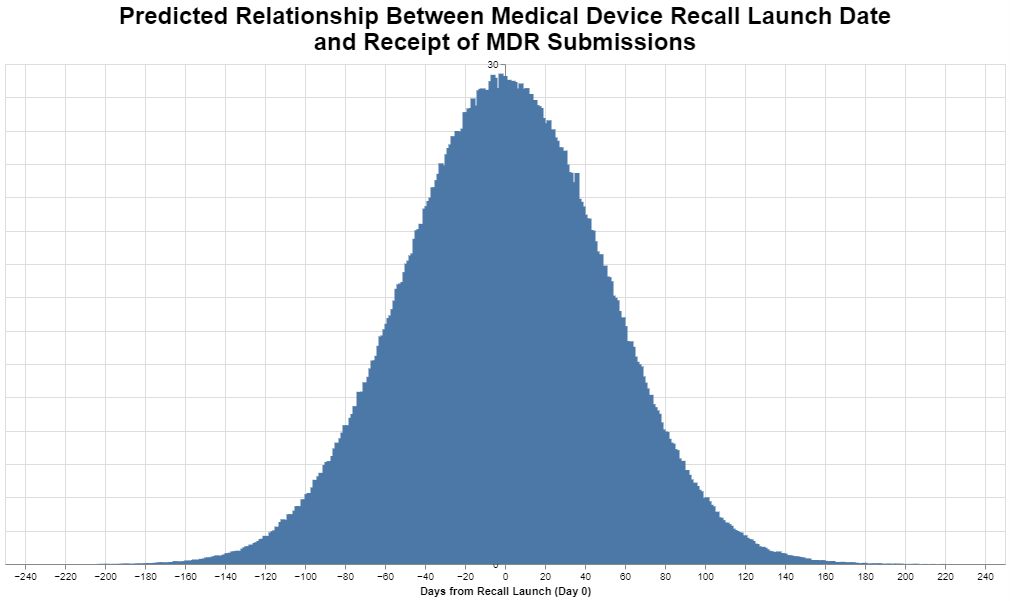
Zero marks the day the recall is launched, and the negative numbers therefore precede that date and the positive numbers follow that date. This is, of course, a perfect bell curve that only software can create based on theory, not likely to be the actual real-world experience.
Colleen wasn’t willing to create a chart of her predicted outcome – which turned out to be smart – but instead simply offered the view that in her experience she often sees a large ramp up of MDRs after the recall is initiated when manufacturers identify a product nonconformance or defect that requires consideration in their risk management program. In other words, she sees the publication of a recall as “causing” MDRs as manufacturers learn to more fully characterize failure modes for product non-conformances
What did the actual data show? Here are the results.
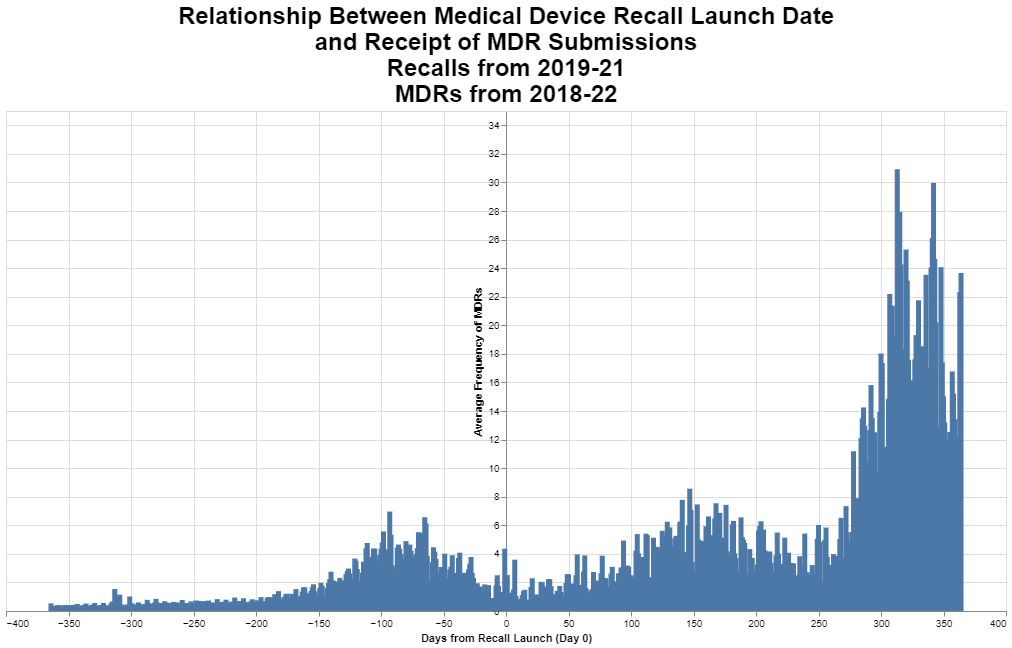
The actual data don’t look a thing like Brad’s theoretical normal curve. The data look like they have three distinct phases including: 1) a bell curve that peaks about three months before the recall is initiated, 2) a curve that peaks maybe five months after the recall is initiated, and then 3) by far the biggest curve peaks almost a year after the recall is initiated. What the hell is that about?
Methodology
Before getting into our analysis, we want to explain how this graph was created. The overarching architecture is to look at the frequency of MDRs by day in reference to the date a recall was initiated. To tie the MDR data with the recall data, we are using K numbers, the numbers that FDA assigns during the course of a 510(k) review. In other words, we are looking for all of the MDRs that share a K number with a given recall. And we are looking 365 days before the initiation of the recall, and 365 days after the initiation of that recall.
It would’ve been cool, and frankly preferable, if we could have then further filtered our analysis to link the product defects cited in the MDR with the quality system nonconformance cited in the recall, but those two databases do not have a common vocabulary for MDR defects and recall root cause. Recall root causes often get reported based on the quality system elements responsible for the recall such as process control, software design, device design, user error, error in labeling, process design, and so forth, where MDR defects or product problems are stated regarding typically the specific failure, such as no device output, battery problem, detachment of device component, material deformation, imprecision, or device alarm system. Thus, we could not come up with a good way to make sure that the MDRs and the recalls were connected other than observing that they both involved the same product as defined by the K number.
We were comforted with the notion that we were looking at very large numbers, namely all of the recalls and all of the MDRs within the one-year time period. Thus, unrelated MDRs would constitute essentially noise when looking at the recalls. But, fortunately, we can see through the noise to the underlying signal, if the signal is strong enough. And not to spill the beans, but the signal seems actually pretty strong.
The dates we looked at for the MDR data necessarily needed to include the year before and the year after the recall data. We settled upon three years of recall data being 2019, 2020 and 2021, to allow us to use MDR data from 2018 through 2022. We appreciate that those were the COVID years and that the COVID years skewed all sorts of things. But we still wanted to use the most recent data rather than going back several years.
To prepare the recall data for this analysis, we were mindful that many manufacturers report what are in essence sub recalls, i.e., several individual recalls that all begin on the same date and all involve the same issue, but they are reported separately for various reasons. As a result, we grouped the sub recalls into one recall for these purposes, if they shared the same: 1) recall initiation date, 2) company, 3) device, 4) root cause, and 5) FDA recall classification. That left us with approximately 4,100 recalls during the relevant time period.
Next, we could obviously only do the analysis if we had a 510(k) or other submission number for a given recall. We therefore further filtered the data to those recalls that had a K number (or a number for a PMA or de novo). Filtering for only those that had a K number left us with just over 2,600 recalls.
Parenthetically, you will note that we typically round to two significant digits. We do this because we remember that in seventh grade science they taught us that you only report as many significant digits as you can based on the precision of your instruments. While we don’t have physical instruments here, we would note that in the filtering we just mentioned, about a third of devices fell out of this analysis only because the K numbers were unknown. That means this analysis must be understood to be merely an approximation.
Out of those 2,600 recalls, there were not quite 2,400 unique K numbers. To explain that number a little bit, while some recalls had two or more K numbers associated with it, we should also add that there were multiple recalls with the same K numbers.
Obviously, we were only concerned with those recalls that had a K number that also had MDRs associated with that K number. That reduced the number further to just over 1,800 recalls during the relevant time period. That in and of itself is a big finding. The implication is that about 800 devices were recalled with no MDRs in the year before or the year after. Obviously, then, many companies decided that they needed to conduct a recall with no adverse MDR experience, perhaps based on complaint data that did not lead to MDRs or their own quality initiatives. Note that we do not take that into account when we calculated the average number of MDRs per recall. We basically left out all those recalls that had zero MDRs connected to them, so the average needs to be understood as the average among those recalls that had MDRs.
The MDR data required less filtering. Over the five years at issue, there were more than 9 million MDRs. At first, we wanted to explore whether the database included double counting, for example, between an initial submission and an update, but we determined that the vast majority – over 99% – were initial submissions of an MDR. When we looked at how many MDRs shared a K number with the recalls, we found that the total was just over 2 million MDRs, a substantial portion of the 9 million MDRs for the relevant time period.
It is not uncommon, in both the recall database and the MDR database, to have more than one K number associated with a given recall or MDR. We chose as our methodology looking to see if any of the listed K numbers for the recall were found among any of the listed K numbers for the MDR.
Interpretation
What did we learn? For one thing, we should acknowledge upfront that all we were doing was looking at patterns. We have not taken any of the steps necessary to establish causal inference. Thus, we can’t, on the basis of our analysis, say anything about what may have caused what.
We were worried that the data would be noisy, in that as explained above we were not able to match the MDRs with the recalls on the basis of a common root cause. In theory, that noise would be randomly dispersed among MDRs across the two years. In other words, by including MDRs that are not connected to the particular recall on the basis of root cause, these disconnected MDRs would occur at any time randomly before or after the recall. So, MDRs on day -116, on day -4, on day 200 would all be equally likely in terms of probability. Those random MDRs not connected to the recalls would basically raise up the whole chart so that the baseline recalls across the entire two years evenly would be higher.
We think it’s interesting that the actual chart is not very noisy. If you look at the area not in the three distinct comps, the valleys are quite low, meaning there are very few MDRs on average in those valleys. That would suggest that even though we didn’t filter the MDRs on the basis of a common root cause with the recalls, that most of the MDRs turned out to be statistically correlated to the recalls. We think that’s interesting.
But the analysis does leave us with some questions that require further probing, sorted by the particular bump.
- 3-month bump (i.e., the bump before the recall).
- Why does the bump seem to resolve itself before the recall even begins? If you look at the 10 days right before the recall, there is a low rate of MDRs.
- Here are a few possible scenarios.
- The bump could mean nothing. While it looks significant, it isn’t statistically significant. It’s just a random sojourn from the median. In other words, the bump could be just statistically random noise that looks a little bit high, but it’s really nothing. Think about that. If that’s the case, then MDR’s really are not a leading indicator of the need for a recall. There is no bump of any sort prior to the recall. MDRs do not indicate the need for a recall. That’s a sobering possibility.
- The bump could mean something. The bump might suggest there is typically some sort of product problem that precedes a recall by about three months. But it resolves most likely on its own, without company intervention, at least, in the form of a recall. In other words, it goes away on its own, and the recall done later to address the bump is while in that sense a result of the bump, it’s superfluous. Unnecessary. It isn’t needed to resolve the issue.
- The bump could mean there’s an issue that arises with the product, the issue is spurred by one root cause, but the recall is done to address a different issue. Maybe the new product problem was brought to light by the MDRs that cause closer examination of the product’s performance. In other words, under this theory, a product problem simply triggers a closer examination by management that causes management to find something worth fixing, even if it turns out not to be the underlying cause of the problem reflected in the MDRs that caught management’s attention.
- The bump is similar to the third-cause fallacy. Even though it seems sequential that the MDRs occur first and then the recall, what if it turns out that there is an event even before the MDRs that leads to both. For example, what if, typically, when a company updates its risk management, such updates may trigger the filing of some new MDRs. Then, in light of that same risk management update, the company conducts a recall that frankly may or may not be connected to the event to trigger the MDRs. The MDRs don’t cause the recall; rather, the update to the risk management approach causes both the MDRs and recalls. The recalls lag the MDRs just because it might take longer to organize the recall, where MDRs can be filed more quickly.
- The bump is, in fact, an early warning signal that leads to the recall, and the resolution prior to the recall is explained this way: there are indeed problems with the products but eventually the customers get fatigued with complaining, i.e., they stop reporting complaints to the company. The customers hear from the company that they’re looking into the issue and decide to hold off on submitting further complaints until they hear something back. The manufacturer’s quality group puts a stop ship in place for a time while they are figuring out what is going on, reducing the volume going out. Only a particular lot at the reporting facilities is affected, and if it’s a consumable it is used up or replaced by a new lot. If it is an instrument of some kind, the initial reporting affected facilities figure out a work around. For example, if an alarm is going off at the wrong time, they just start ignoring an alarm, or if an audible alarm doesn’t work but a light does, they just check the light and know not to wait for the audible alarm, and don’t complain anymore. Service techs start going out and servicing devices as part of maintenance as the initial complaints come in, but this hasn’t reached the point where quality / regulatory have decided to initiate a recall (basically, one arm of the company is fixing the issues as part of service/maintenance contracts, but it isn’t clear yet to regulatory / quality that there is a problem. Viola, the MDRs recede before the recall is announced.
- As we said, these are just theories and we have no idea which, if any, is right.
- 5-month bump.
- Frankly, any bump after the initiation of the recall is a little bit troubling. It means that on average after a company conducts a recall, MDRs go up. And that happens after roughly 5 months.
- Here are a few possible alternate scenarios.
- As always in these sorts of analyses, it might be nothing. It might be a statistical anomaly. This would be the most comforting conclusion because it would mean that the recall worked just fine on average.
- The 5-month bump is attributed to increased general awareness among customers of the new failure mode, customers who then submit more complaints that ultimately get turned into MDRs. Some customers see the recall letter notifying them of a problem, so they’re more likely to detect and report to the company to get a replacement product. As company techs go out, now as part of a recall, to repair instruments, they are having more interactions with customers about problems and submitting more complaints. But the 5-month bump also seems a bit delayed for that. Even Colleen expected the effect to follow more closely on the heels of the recall event. And, whatever this is, it seems to resolve itself before leading to the third bump.
- The 5-month bump might happen because on average the recall was ineffective and so there are generally other events that occur. This is obviously the most disconcerting possibility. But it still seems odd that the bump waits five months. Why did the rates go down initially after the recall?
- The 5-month bump might be caused by the recall in a different way. Perhaps the recall caused companies to take a closer look at their risk management procedures and maybe they even change the threshold for reporting events to FDA. And that change in reporting goes into effect about five months on average after the recall.
- Intuitively, we like to believe that the recall somehow caused the increase in MDRs in a positive way, that the recall itself was effective but it led to either greater awareness or a change in procedure that produced the higher MDRs. But that isn’t necessarily the case.
- One year bump.
- This is the one that really confounds us, both because it’s a year later and because it’s so much bigger than the other two bumps.It seems to be an awful long time if it is the result of the publicity associated with the recall or the change in procedures which we theorized might explain the five-month bump, but that is still possible.
- Here are a few possible alternate scenarios.
- The company does what it’s going to do on its own, and that produces a bump at 5 months. But then FDA gets involved, and FDA typically has different or more ideas about changing the risk management or the MDR thresholds, and closer to a year, the company implements changes at the request or encouragement of FDA. We suppose this explanation could also apply to the 5-month bump, but on our experience, it often takes FDA longer to make these “suggestions.”
- Lots of times it takes multiple follow-ups to get customers to reply to a recall letter. The third spike might be the usual second or third time following up where the customer finally acknowledges there is a problem and the submits complaints / feedback to the company.
- Also, competitors getting wind of the recall are spreading the news too (to try and switch clients), but that takes time. Regardless of whoever is bringing it to their attention (company or its competitors), the recall is raising awareness and makes it more likely for MDR reportable complaints to be forwarded to the company as customers are trying to get replacement product or service or money back.
- Recalls are expanded based on additional information being gathered to encompass additional lots of product, which in turn prompts more complaints that become MDRs.
- Theories not specific to a particular bump.
- While the MDR analysis covers 5 years, in our observation the COVID pandemic impacted just about everything including FDA practices and how medical device users operate. Our chart could be a COVID-19 artifact. But just to repeat the methodology a little bit, for a recall that was initiated on January 22, 2019, this data looks at the MDRs that occurred one year before that and one year after that. For a recall that began on June 17, 2020, likewise the MDR recall are from the data one year before and one year after. In a sense it’s like a moving average. If the data were highly unusual in, say, November 2020, that would be reasonably smoothed over by recalls that occurred prior to that and after that. But again, there’s no doubt that COVID 19 had some effect and we would need to choose earlier years to see if there is any significant difference. Maybe that’s a future post. It’s more work than you might think.
- Recall from the methodology that we filtered to those recalls that had a K number. We tried to think through whether excluding recalls that did not have a K number might produce something quirky here. Some recalls without K numbers might be because the device was exempt, while other recalls without K numbers might simply be because no one knew the number. FDA typically considers the number of MDRs filed when judging whether to exempt a product, so we are thinking that most of these that were excluded were excluded because of simply human omission of the K number. So, we simply have to note that it’s a possibility that that exclusion has somehow skewed the data to produce this quirky result.
On the whole, our chart certainly does not make a compelling case that MDRs are a good leading indicator for recalls. Manufacturers seem plainly motivated by other factors perhaps such as investigating root cause of quality problems including updating their risk management files with new and emerging post-market data insights.
Conclusions
Although this analysis gives Colleen the opportunity to rub Brad’s nose in the error of his theoretical predictions, it doesn’t really provide answers, just more questions. The one thing that seems clear is that it doesn’t really support MDRs as a useful data set for predicting the likelihood of future recalls. The signal there seems pretty weak as that is clearly the least significant of the three bumps in the timeline.
For industry, this analysis mostly confirms that recalls are expensive, in that they could well prompt an increase in the number of adverse events being reported. In the same breath, we note that these are MDRs that the manufacturers have determined need to be reported, so there is at least the possibility that they have public health significance, even as a lagging indicator.
For FDA, this analysis draws into question whether the MDR process is really serving any important function, as it does not seem to be a particularly good leading indicator that can be used to predict the likelihood of a future recall. The value of the MDR data to public health appears limited based on this analysis, as it as at best a lagging indicator. Its greatest value is really to FDA to help the agency prepare for field investigations, or perhaps compare post-market experiences between competitive products.
The FDA data analyzed for this blog post are publicly available through an API at https://open.fda.gov/
* * * *
The Unpacking Averages® blog series digs into FDA’s data on the regulation of medical products, going deeper than the published averages. The opinions expressed in this publication are those of the author(s). Subscribe to this blog for email notifications.
Blog Editors
Authors
- Member of the Firm